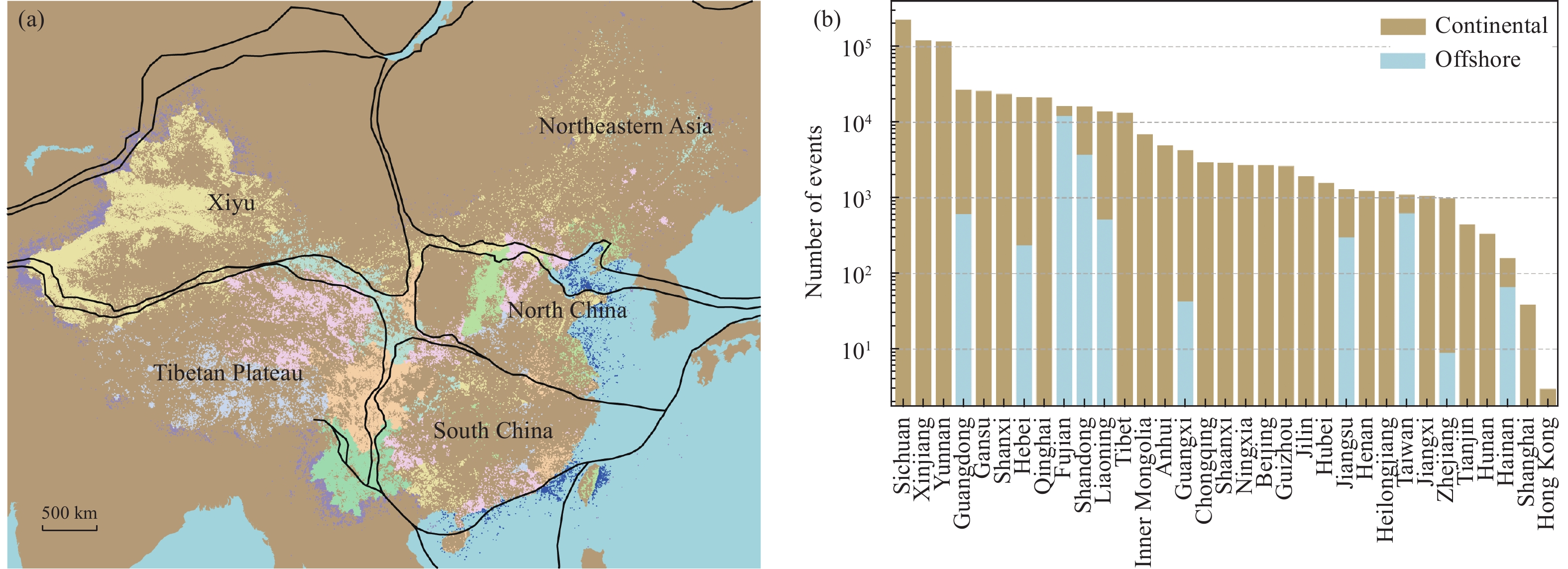
Citation: | Zhu J, Li ZF and Fang LH (2023). USTC-Pickers: a Unified Set of seismic phase pickers Transfer learned for China. Earthq Sci 36(2): 95–112,. DOI: 10.1016/j.eqs.2023.03.001 |
Current popular deep learning seismic phase pickers like PhaseNet and EQTransformer suffer from performance drop in China. To mitigate this problem, we build a unified set of customized seismic phase pickers for different levels of use in China. We first train a base picker with the recently released DiTing dataset using the same U-Net architecture as PhaseNet. This base picker significantly outperforms the original PhaseNet and is generally suitable for entire China. Then, using different subsets of the DiTing data, we fine-tune the base picker to better adapt to different regions. In total, we provide 5 pickers for major tectonic blocks in China, 33 pickers for provincial-level administrative regions, and 2 special pickers for the Capital area and the China Seismic Experimental Site. These pickers show improved performance in respective regions which they are customized for. They can be either directly integrated into national or regional seismic network operation or used as base models for further refinement for specific datasets. We anticipate that this picker set will facilitate earthquake monitoring in China.
Earthquake detection and phase arrival picking are fundamental for earthquake monitoring. Earthquake detectability in a region, often measured by magnitude of completeness, is largely determined by the capability to pick accurate seismic phases in presence of noise. Missing small earthquakes may reduce the resolution to image source processes and subsurface structures. Also, the accuracy of phase arrival determination directly impacts the reliability of derived earthquake locations and velocity models.
For a long time, seismic phase has been manually picked by analysts and assisted with automatic pickers, such as short-term-average/long-term-average ratio (STA/LTA) (Allen, 1978, 1982). However, pickers like STA/LTA oversimplify rich waveform characteristics and their performances tend to deteriorate in noisy data. Recently, deep learning phase pickers, such as PhaseNet (Zhu WQ and Beroza, 2019), EQTransformer (Mousavi et al., 2020), Generalized phase detection (GPD, Ross et al. 2018), CPIC (Zhu LJ et al., 2019), have been revolutionizing the way of seismic phase picking. Differing from traditional pickers, deep learning pickers learn implicit waveform features from a large number of labelled picks. They are demonstrated to outperform traditional phase pickers and approaches the accuracy of manual picking.
Unfortunately, deep learning pickers often suffer from a so-called “generalizability” problem. That is, although these pickers mostly perform better than traditional methods (e.g., STA/LTA), their performances tend to drop in regions other than where they are trained (Jiang C et al., 2021; Lapins et al., 2021). Also, training a deep-learning picker from scratch is expensive and sometimes impossible, as not all regions have sufficient manual labels. Comparatively, transfer learning only requires a small set of labelled data and is advantageous to adapt existing pickers for different regions (Zhu LJ et al., 2019; Chai CP et al., 2020; Lapins et al., 2021).
Here, we use transfer learning to build a unified set of seismic phase pickers for different levels of use in China. As follows, we first introduce the data and methods to obtain these pickers. Then we evaluate their performances in the regions they are customized for. Finally, we discuss their usages and analyze the performance variations across China.
We use a U-Net architecture the same as PhaseNet owing to its proved success in phase picking tasks (Zhu WQ and Beroza, 2019; Münchmeyer et al., 2022; Liao SR et al., 2021). A China dataset, DiTing, compiled by Zhao M et al. (2023) is used to train the network and its subsets are used to customize pickers for different regions using transfer learning. The DiTing dataset contains 787,010 local-to-regional earthquakes with 2,734,748 P and S picks recorded by the China Earthquake Networks in 2008–2020 (Figure 1).
Specifically, we first train a model with the entire DiTing dataset to obtain a picker that is generally suitable for entire China, named as CN. Then, we split the DiTing data into five geological subregions and use CN as the base model for transfer learning. These regional pickers can be used in each area when the performance of CN is not satisfactory. We further divide the DiTing data into 33 provinces and build the customized pickers for them (Macao is excluded because of no earthquakes there). The training dataset keeps the same in training the general picker, fine-tuning for subregions and provinces. The provincial pickers use their parent regional pickers as base pickers for transfer learning (Figure 2). The potential users of the provincial pickers are permanent network operators in each province or real time earthquake processing system, such as RISP (Liao SR et al., 2021) and EEW (Li ZF et al., 2018; Allen and Melgar, 2019).
Finally, we build two other pickers specifically for the Capital area and the China Seismic Experimental Site (CSES), which are critical areas of earthquake monitoring in China. The Capital area picker is fine-tuned with data from Beijing, Tianjin and Hebei. The CSES picker is fine-tuned with data from Sichuan, Yunnan and Guizhou. The data division and data statistics in each subregion are provided in Table 1 and Figure 1b. We collectively call the 41 pickers as USTC-pickers (a Unified Set of pickers Transfer learned for China).
Our pickers are built using a toolbox for machine learning in seismology called SeisBench (Woollam et al., 2022). SeisBench integrates several popular deep-learning phase pickers and benchmark datasets as well as a convenient environment for deep and transfer learning. In training the picker for entire China, we randomly initiate the U-Net model and train it with the DiTing dataset from scratch. In transfer learning of other pickers, we adopt a strategy called fine-tuning, that is, set all the parameters in the base picker trainable and continue training the base picker with new data. For each training task, we split the corresponding data into training (70%), validation (10%), and test (20%) sets. The training/validation/test data of all provinces form the training/validation/test data of respective subregions, respectively; similarly, the training/validation/test data of all subregions form the training/validation/test data of the whole DiTing data for the CN picker, respectively. Notice that when comparing different pickers in a given region, the test set remains the same. This ensures that the test set has never been seen by any picker before model evaluation. We randomly shift the waveform window to unfix the positions of P/S phases (Zhu WQ and Beroza, 2019; Zhu WQ et al., 2020). No other data augmentation techniques are used.
We use the Adam solver (Kingma and Ba, 2015) for optimization and a learning rate of 0.001. Early stopping is used to obtain an optimal model when the validation loss fails to decrease in five consecutive epochs. All three-component waveforms include both the P and S phases. They have been sampled at 50 Hz (Zhao M et al., 2023) and cropped to 60 s so that the input of USTC-pickers has a dimension of 3×3001. We further detrend and normalize the three-component waveforms by their maximal standard deviation. We set the pick labels as Gaussian windows with a standard deviation of 1 s to encompass manual picking errors. This slightly broader Gaussian shape than that used in Zhu WQ and Beroza (2019) is determined by trial-and-error to stabilize the training process. A broader Gaussian mask can help mitigate the negative effects of manual labelling errors. We apply a peak-detection algorithm (Duarte and Watanabe, 2021) to the P and S probability functions to get the P and S arrival times. This algorithm contains three key parameters, a probability threshold for P phases (set as 0.3), a probability threshold for S phases (0.3) and a minimal separation between two consecutive P or S picks (50 sample points).
Region | Abbreviation | Provinces | Events | P/S pick pairs |
China | CN | All DiTing data | 715,806 | 2,498,982 |
Xiyu | XY | Xinjiang, Inner Mongolia, Gansu | 154,633 | 644,388 |
Northeastern Asia | NA | Heilongjiang, Jilin, Liaoning, Inner Mongolia, Beijing, Tianjin, Hebei | 48,805 | 280,761 |
Tibetan Plateau | TP | Yunnan, Sichuan, Ningxia, Gansu, Tibet, Qinghai | 409,179 | 1,187,626 |
North China | NC | Ningxia, Shanghai, Beijing, Tianjin, Anhui, Shandong, Shanxi, Hebei, Henan, Jiangsu, Shaanxi | 77,799 | 436,241 |
South China | SC | Yunnan, Sichuan, Anhui, Zhejiang, Hainan, Hubei, Hunan, Fujian, Guizhou, Chongqing, Shaanxi, Taiwan, Hong Kong, Guangxi, Guangdong, Jiangxi | 411,905 | 1,210,775 |
Capital area | Capital | Beijing, Tianjin, Hebei | 24,811 | 173,038 |
China Seismic Experimental Site | CSES | Sichuan, Yunnan, Guizhou | 348,266 | 950,826 |
Note: Some provinces are assigned to more than one tectonic block as they somewhat cover the boundary of blocks. |
To evaluate the pickers’ performance, we first define the picking residual as Δt=tAI−tmanual, where tAI and tmanual are the arrival time provided by USTC-pickers and analysts, respectively. This picking residual enables us to evaluate the systematic shift/bias of the AI picks with its mean, which is unavailable with an absolute residual (e.g., |tAI−tmanual|). The standard deviation of Δt serves as a measure of overall pick errors.
In addition to the picking residual, we define various metrics to evaluate the performance of the pickers:
(1) true positive (TP): the AI pick with |Δt| ≤0.6 s and probability >0.3;
(2) false positive (FP): the AI pick with |Δt| >0.6 s and probability >0.3; note FPs and TPs sum to be all the positive AI picks;
(3) false negative (FN): the true pick which has no TP within the tolerance range (i.e., tmanual±0.6 s); note FNs and TPs sum to be all the true picks;
(4) true negative (TN): the sample point of noise predicted as noise.
(5) precision: P=TP/(TP+FP),
(6) recall: R=TP/(TP+FN),
(7) F1 score: F1=2×P×R/(P+R).
These definitions are similar to those in the previous literature (Zhu WQ and Beroza, 2019; Mousavi et al., 2020; Jiang C et al., 2021). The difference lies in that the TP has picking residual within ±0.6 s and probabilities more than 0.3. We choose 0.6 s as an error tolerance due to the lower sampling rate of the DiTing (50 Hz) compared to PhaseNet and EQTransformer data (100 Hz). The probability threshold of 0.3 is a default value used in the GitHub repositories of PhaseNet and SeisBench. We provide some example picks with probabilities near 0.3 to support the choice of this threshold (Figure S1).
Table 2 shows comparison between the CN picker and the original PhaseNet which was trained with northern California data (called the NoCal picker hereafter, Zhu WQ and Beroza, 2019). The CN picker outperforms the NoCal PhaseNet in F1-score by 6.7% for P phases and 8.2% for S phases. The improvement in recall is particularly significant. The CN picker reduces the mean residual by 84 ms for P phases and 155 ms for S phases, meaning a smaller systematic bias. The mean absolute error of picking residuals is reduced by 17 ms for P phases and 34 ms for S phases. The standard deviation of the picking residuals of the CN picker is slightly larger than that of the NoCal PhaseNet, likely because we use a broader Gaussian mask window to stabilize the training process. Generally, the CN picker performs well in the test dataset.
Figure 3 shows comparison in precision, recall and F1-score for different pickers in five tectonic blocks and two special regions. The regional pickers outperform the CN picker by a small amount, i.e., the F1-score increases by 0.5%–2.2% for P phases and 0.9%–2.6% for S phases. This small increase can be attributed to potentially different earthquake characteristics in different regions and the region-customized pickers further adapt to these differences.
Evaluation metrics | Phase | CN | NoCal |
Precision | P | 87.4% | 85.7% |
S | 80.2% | 77.1% | |
Recall | P | 89.8% | 78.5% |
S | 82.1% | 69.0% | |
F1-sccore | P | 88.6% | 81.9% |
S | 81.1% | 72.9% | |
MAE(Δt) (ms) | P | 123.491 | 140.586 |
S | 190.255 | 224.024 | |
μ(Δt) (ms) | P | 28.197 | 112.159 |
S | 32.803 | 187.435 | |
σ(Δt) (ms) | P | 191.441 | 179.795 |
S | 272.783 | 245.791 | |
Note: The NoCal picker is the original PhaseNet, which was trained with Northern California data. NoCal is used hereafter. MAE is the mean absolute error of picking residuals. |
Figure 4 and Table 3 compare the performance of the provincial pickers with the NoCal pickers nationwide. Significant regional variations are observed, partly due to training data volume in each province (Figure 5). We note a weak correlation between the F1-scores and the training data sizes. Specifically, above 10,000 samples seem required to achieve high F1-scores (say, >82% for P and >79% for S). For provinces with less than 10,000 samples, the variations are much wider. This result suggests 10,000 samples is a probable threshold to train the model sufficiently, which could be related to the model size (that is, the number of free parameters). Apart from the impact of data volume, regional data characteristics may also play a role in performance variations. With similar data volume above 10,000 samples, the F1-scores of P phase vary within 82%–94%. The F1-scores of S phase vary even more. Especially, Tibet and Xinjiang, although with relatively large data volumes, have unusually low F1-scores of S phase, which will be discussed in the following section.
No. | Province | CN picker | Block picker | Provincial picker | |||||
Phase P | Phase S | Phase P | Phase S | Phase P | Phase S | ||||
1 | Sichuan | 88.8% | 79.9% | 89.6% | 81.3% | 89.9% | 81.8% | ||
2 | Xinjiang | 86.7% | 73.3% | 88.2% | 75.1% | 88.1% | 75.2% | ||
3 | Yunnan | 89.4% | 82.5% | 90.3% | 83.9% | 90.2% | 83.7% | ||
4 | Guangdong | 82.2% | 83.3% | 84.7% | 85.0% | 86.2% | 86.0% | ||
5 | Gansu | 91.6% | 85.7% | 92.1% | 86.6% | 92.2% | 86.7% | ||
6 | Shanxi | 85.7% | 86.0% | 88.8% | 89.0% | 89.2% | 89.3% | ||
7 | Hebei | 83.3% | 81.9% | 85.6% | 84.4% | 85.5% | 84.3% | ||
8 | Qinghai | 93.2% | 84.6% | 93.6% | 85.2% | 93.8% | 86.8% | ||
9 | Fujian | 82.2% | 84.9% | 85.0% | 86.3% | 86.4% | 87.1% | ||
10 | Shandong | 91.3% | 88.9% | 90.6% | 88.9% | 91.9% | 90.2% | ||
11 | Liaoning | 88.9% | 86.3% | 90.9% | 89.0% | 91.8% | 89.8% | ||
12 | Tibet | 82.5% | 66.1% | 83.5% | 67.1% | 84.3% | 68.7% | ||
13 | Inner Mongolia | 85.5% | 80.0% | 87.1% | 81.0% | 87.5% | 82.1% | ||
14 | Anhui | 89.3% | 87.0% | 89.8% | 87.1% | 90.5% | 88.0% | ||
15 | Guangxi | 73.3% | 68.7% | 74.9% | 70.1% | 75.4% | 71.0% | ||
16 | Chongqing | 86.2% | 72.9% | 86.6% | 72.9% | 86.1% | 74.8% | ||
17 | Shaanxi | 89.2% | 84.8% | 90.3% | 84.8% | 91.3% | 86.2% | ||
18 | Ningxia | 91.6% | 79.0% | 92.0% | 79.3% | 92.2% | 79.4% | ||
19 | Beijing | 78.5% | 79.7% | 82.4% | 82.7% | 82.1% | 82.9% | ||
20 | Guizhou | 76.6% | 72.2% | 77.3% | 72.6% | 77.2% | 73.1% | ||
21 | Jilin | 86.4% | 85.2% | 87.9% | 86.5% | 89.3% | 88.6% | ||
22 | Hubei | 87.1% | 75.8% | 88.2% | 74.7% | 88.4% | 74.8% | ||
23 | Jiangsu | 85.4% | 77.5% | 85.3% | 77.4% | 86.2% | 80.1% | ||
24 | Henan | 86.8% | 80.6% | 87.9% | 82.0% | 87.8% | 82.5% | ||
25 | Heilongjiang | 88.4% | 80.6% | 88.8% | 82.3% | 89.6% | 82.3% | ||
26 | Taiwan | 91.0% | 75.9% | 91.2% | 78.4% | 90.8% | 79.8% | ||
27 | Jiangxi | 77.0% | 70.1% | 79.4% | 72.8% | 81.1% | 72.7% | ||
28 | Zhejiang | 85.8% | 85.2% | 86.8% | 85.6% | 87.9% | 86.3% | ||
29 | Tianjin | 85.1% | 76.7% | 87.3% | 78.6% | 87.1% | 79.2% | ||
30 | Hunan | 80.4% | 74.2% | 82.0% | 74.9% | 81.1% | 72.9% | ||
31 | Hainan | 81.6% | 81.1% | 84.4% | 82.8% | 86.2% | 85.7% | ||
32 | Shanghai | 81.6% | 87.5% | 88.3% | 91.1% | 88.2% | 87.7% | ||
33 | Hong Kong | 70.6% | 23.5% | 75.0% | 40.0% | 73.7% | 40.0% | ||
Note: The number of earthquake events gradually decreases from Sichuan to Hong Kong. The block pickers for Yunnan, Hebei, Beijing, Hunan, Shanghai and Hong Kong are TP, NC, NC, SC, NC and SC, respectively. |
Figure 6 shows comparison in precision, recall and F1-score for different pickers in 33 provinces. The provincial pickers show marginal improvement (mostly within 2%) in F1-score compared to the regional pickers. Generally, the enhancements are mostly for the low signal-to-noise ratio waveforms (Figure 7). These incremental changes indicate gradual adaption to local earthquake characteristics by transfer learning from the parent pickers. Three exceptions exist in Yunnan, Hebei and Beijing where the regional and provincial pickers perform comparably. Three other exceptions are Hunan, Shanghai, and Hong Kong, where data sizes are very small (Figure 1) and hence both model training and evaluation are likely unstable (Figures S6 and S7).
Using the PhaseNet architecture and the DiTing data, we have built a picker generally suitable for entire China and transferred it to different regions and provinces. The 41 pickers are publicly available via the link in Data Availability. One can use the PyTorch framework (Paszke et al., 2019) to load and utilize these pickers. Although not required, as a best practice, implementing them within SeisBench will provide most of the power and convenience.
Ideally, our seismological community looks for an optimal model that works universally for all seismic data, which is convenient and elegant. This pursuit is manifested by continuous efforts on looking for better network architectures as well as more training data in the past five years (Ross et al. 2018; Zhu WQ and Beroza, 2019; Zhu LJ et al., 2019; Mousavi et al., 2020; Jiang C et al., 2021; Liao SR et al., 2021; Münchmeyer et al., 2022). Our motivation and approach of this research are based on a different philosophy. We recognize that earthquake characteristics vary widely as a combined result of different regional structures, source, site effect, environmental noise, instrument types and array settings. Hence, seismic data tend to have strong local imprints. Deep learning pickers inevitably suffer from limited generalizability — their performances tend to drop to various extents when applied to regions other than where the pickers are trained (Jiang C et al., 2021; Lapins et al., 2021). Hence, rather than seeking a universal but likely mediocre picker, it seems preferrable to seek an optimal picker customized for each dataset when possible. As we have shown, transfer learning offers an economical way to achieve so.
As the regional and provincial data are already included in the national data, the data characteristics are not so distinctive, leading to only minor improvement of the transferred pickers over the CN picker. One can anticipate more significant improvement from transfer learning between two more different datasets (Figures S2−S7). Such a small amount of improvement raises the question of whether it is worth applying transfer learning to regional and provincial networks. We argue that the potential users of these pickers such as provincial network operators may gain substantial benefit from small improvement in the long run, without increasing their operational burden (compared to using other models). Moreover, transfer learning allows more economical construction of these pickers than training from scratch as transfer learning requires less labelled data and often converges faster.
Besides the diminishing benefit, the acceptable operational complexity is another determinant of when to stop transferring. One may ask what if we further transfer for individual seismic stations? That might improve the performances on individual stations by a tiny amount. However, it would likely bring too much operational burden as provincial operators need to navigate among tens to hundreds of pickers.
The unusually low F1-scores of S phase in Tibet and Xinjiang are likely due to more farther earthquakes in the regions compared to national average and that the F1-scores of S phase decrease with epicentral distance (Figure 8). This distance dependence is consistent with poor performances of cross-domain transfer learning between the regional and teleseismic distances (Münchmeyer et al., 2022). We speculate several reasons for this distance dependence. First, longer epicentral distance generally leads to lower SNRs. Second, the model takes a fixed window length (60 s) as input and drops part of S coda for long epicentral distances. Third, there are potential interferences from Sn phases (Zhao M et al., 2023). In addition, the even worse performance in Tibet (Figure 8b) could be associated with the complex geologic settings beneath Tibetan Plateau (Shapiro et al., 2004; Bao XW et al., 2015).
The pickers generally perform well on the permanent seismic networks by which the DiTing data are recorded. Which picker to use will be determined by station locations, as well as the trade-off between requirement for picking accuracy and acceptable operational complexity. As we have shown, the improvement from the CN picker to the regional pickers are within 3% and the improvement from the regional pickers to provincial pickers are within 2%, whereas the number of models increases from 1 to 5 and from 5 to 33, respectively. For temporary networks in a particularly local scale, with different instrument types (e.g., short-period, nodal sensors) and/or with sampling rates other than 50 Hz, the performance of USTC-pickers is likely to drop to some extent. In this case, we suggest applying transfer learning again to further customize the pickers for the data. On the other hand, we will keep update the USTC-pickers when new data and improved model architectures are available. We envision that adoption of these deep learning pickers to permanent and temporary seismic networks will facilitate routine earthquake monitoring and seismological research in China.
This research was supported by the National Key R&D Program of China (No. 2021YFC3000700) and the Special Fund of the Institute of Geophysics, China Earthquake Administration (Nos. DQJB22X08 and DQJB21Z05). We thank the DiTing team and China Earthquake Networks Center for providing the DiTing dataset. Particularly, we thank Li Sun, Zhuowei Xiao and Ming Zhao for help in accessing the dataset. We also thank EIC Xiaodong Song and two anonymous reviewers for their detailed and constructive comments to improve this article. Jun Zhu thanks the SeisBench team and Yiyu Ni for answering various questions regarding the use of SeisBench.
Allen R (1982). Automatic phase pickers: their present use and future prospects. Bull Seismol Soc Am 72(6B): S225–S242 https://doi.org/10.1785/BSSA07206B0225.
|
Allen RM and Melgar D (2019). Earthquake early warning: advances, scientific challenges, and societal needs. Annu Rev Earth Planet Sci 47: 361–388 https://doi.org/10.1146/annurev-earth-053018-060457.
|
Allen RV (1978). Automatic earthquake recognition and timing from single traces. Bull Seismol Soc Am 68(5): 1521 – 1532 https://doi.org/10.1785/BSSA0680051521.
|
Bao XW, Sun XX, Xu MJ, Eaton DW, Song XD, Wang LS, Ding ZF, Mi N, Li H, Yu DY, Huang ZC and Wang P (2015). Two crustal low-velocity channels beneath SE Tibet revealed by joint inversion of Rayleigh wave dispersion and receiver functions. Earth Planet Sci Lett 415: 16 – 24 https://doi.org/10.1016/j.jpgl.2015.01.020.
|
Chai CP, Maceira M, Santos-Villalobos HJ, Venkatakrishnan SV, Schoenball M, Zhu WQ, Beroza GC, Thurber C and EGS Collab Team (2020). Using a deep neural network and transfer learning to bridge scales for seismic phase picking. Geophys Res Lett 47(16): e2020GL088651 https://doi.org/10.1029/2020GL088651.
|
Duarte M, and Watanabe RN (2021). Notes on scientific computing for biomechanics and motor control. https://zenodo.org/record/4599319#.Y0y1d8hSEw8. Accessed 11 March 2021.
|
Jiang C, Fang LH, Fan LP and Li BR (2021). Comparison of the earthquake detection abilities of PhaseNet and EQTransformer with the Yangbi and Maduo Earthquakes. Earthq Sci 34(5): 425 – 435 https://doi.org/10.29382/eqs-2021-0038.
|
Kingma DP and Ba J (2015). Adam: A method for stochastic optimization. In: Proceedings of the 3rd International Conference on Learning Representations. May 7-9, 2015, San Diego, CA, USA, arXiv e-prints.
|
Lapins S, Goitom B, Kendall JM, Werner MJ, Cashman KV and Hammond JOS (2021). A little data goes a long way: automating seismic phase arrival picking at Nabro volcano with transfer learning. J Geophys Res: Solid Earth 126(7): e2021JB021910 https://doi.org/10.1029/2021JB021910.
|
Li ZF, Meier MA, Hauksson E, Zhan ZW and Andrews J (2018). Machine learning seismic wave discrimination: application to earthquake early warning. Geophys Res Lett 45(10): 4773 – 4779 https://doi.org/10.1029/2018GL077870.
|
Liao SR, Zhang HC, Fan LP, Li BR, Huang LZ, Fang LH and Qin M (2021). Development of a real-time intelligent seismic processing system and its application in the 2021 Yunnan Yangbi MS6.4 earthquake. Chin J Geophys 64(10): 3632 – 3645 https://doi.org/10.6038/cjg2021O0532 (in Chinese with English abstract).
|
Mousavi SM, Ellsworth WL, Zhu WQ, Chuang LY and Beroza GC (2020). Earthquake transformer—an attentive deep-learning model for simultaneous earthquake detection and phase picking. Nat Commun 11(1): 3952 https://doi.org/10.1038/s41467-020-17591-w.
|
Münchmeyer J, Woollam J, Rietbrock A, Tilmann F, Lange D, Bornstein T, Diehl T, Giunchi C, Haslinger F, Jozinović D, Michelini A, Saul J and Soto H (2022). Which picker fits my data? A quantitative evaluation of deep learning based seismic pickers. J Geophys Res: Solid Earth 127(1): e2021JB023499 https://doi.org/10.1029/2021JB023499.
|
Paszke A, Gross S, Massa F, Lerer A, Bradbury J, Chanan G, Killeen T, Lin ZM, Gimelshein N, Antiga L, Desmaison A, Köpf A, Yang EZ, DeVito Z, Raison M, Tejani A, Chilamkurthy S, Steiner B, Fang L, Bai JJ, and Chintala S (2019). PyTorch: an imperative style, high-performance deep learning library. In: Advances in Neural Information Processing Systems. The 33rd Conference on Neural Information Processing Systems (NeurIPS), Vancouver, BC, Canada, arXiv e-prints.
|
Ross ZE, Meier MA, Hauksson E and Heaton TH (2018). Generalized seismic phase detection with deep learning. Bull Seismol Soc Am 108(5A): 2894 – 2901 https://doi.org/10.1785/0120180080.
|
Shapiro NM, Ritzwoller MH, Molnar P and Levin V (2004). Thinning and flow of Tibetan crust constrained by seismic anisotropy. Science 305(5681): 233 – 236 https://doi.org/10.1126/science.1098276.
|
Woollam J, Münchmeyer J, Tilmann F, Rietbrock A, Lange D, Bornstein T, Diehl T, Giunchi C, Haslinger F, Jozinović D, Michelini A, Saul J and Soto H (2022). SeisBench—a toolbox for machine learning in seismology. Seismol Res Lett 93(3): 1695 – 1709 https://doi.org/10.1785/0220210324.
|
Zhao M, Xiao ZW, Chen S and Fang LH (2023). DiTing: a large-scale Chinese seismic benchmark dataset for artificial intelligence in seismology. Earthq Sci 36(2): 84 – 94 https://doi.org/10.1016/j.eqs.2022.01.020.
|
Zhu LJ, Peng ZG, McClellan J, Li CY, Yao DD, Li ZF and Fang LH (2019). Deep learning for seismic phase detection and picking in the aftershock zone of 2008 MW7.9 Wenchuan Earthquake. Phys Earth Planet Inter 293: 106261 https://doi.org/10.1016/j.pepi.2019.05.004.
|
Zhu WQ and Beroza GC (2019). PhaseNet: a deep-neural-network-based seismic arrival-time picking method. Geophys J Int 216(1): 261– 273 https://doi.org/10.1093/gji/ggy423.
|
Region | Abbreviation | Provinces | Events | P/S pick pairs |
China | CN | All DiTing data | 715,806 | 2,498,982 |
Xiyu | XY | Xinjiang, Inner Mongolia, Gansu | 154,633 | 644,388 |
Northeastern Asia | NA | Heilongjiang, Jilin, Liaoning, Inner Mongolia, Beijing, Tianjin, Hebei | 48,805 | 280,761 |
Tibetan Plateau | TP | Yunnan, Sichuan, Ningxia, Gansu, Tibet, Qinghai | 409,179 | 1,187,626 |
North China | NC | Ningxia, Shanghai, Beijing, Tianjin, Anhui, Shandong, Shanxi, Hebei, Henan, Jiangsu, Shaanxi | 77,799 | 436,241 |
South China | SC | Yunnan, Sichuan, Anhui, Zhejiang, Hainan, Hubei, Hunan, Fujian, Guizhou, Chongqing, Shaanxi, Taiwan, Hong Kong, Guangxi, Guangdong, Jiangxi | 411,905 | 1,210,775 |
Capital area | Capital | Beijing, Tianjin, Hebei | 24,811 | 173,038 |
China Seismic Experimental Site | CSES | Sichuan, Yunnan, Guizhou | 348,266 | 950,826 |
Note: Some provinces are assigned to more than one tectonic block as they somewhat cover the boundary of blocks. |
Evaluation metrics | Phase | CN | NoCal |
Precision | P | 87.4% | 85.7% |
S | 80.2% | 77.1% | |
Recall | P | 89.8% | 78.5% |
S | 82.1% | 69.0% | |
F1-sccore | P | 88.6% | 81.9% |
S | 81.1% | 72.9% | |
MAE(Δt) (ms) | P | 123.491 | 140.586 |
S | 190.255 | 224.024 | |
μ(Δt) (ms) | P | 28.197 | 112.159 |
S | 32.803 | 187.435 | |
σ(Δt) (ms) | P | 191.441 | 179.795 |
S | 272.783 | 245.791 | |
Note: The NoCal picker is the original PhaseNet, which was trained with Northern California data. NoCal is used hereafter. MAE is the mean absolute error of picking residuals. |
No. | Province | CN picker | Block picker | Provincial picker | |||||
Phase P | Phase S | Phase P | Phase S | Phase P | Phase S | ||||
1 | Sichuan | 88.8% | 79.9% | 89.6% | 81.3% | 89.9% | 81.8% | ||
2 | Xinjiang | 86.7% | 73.3% | 88.2% | 75.1% | 88.1% | 75.2% | ||
3 | Yunnan | 89.4% | 82.5% | 90.3% | 83.9% | 90.2% | 83.7% | ||
4 | Guangdong | 82.2% | 83.3% | 84.7% | 85.0% | 86.2% | 86.0% | ||
5 | Gansu | 91.6% | 85.7% | 92.1% | 86.6% | 92.2% | 86.7% | ||
6 | Shanxi | 85.7% | 86.0% | 88.8% | 89.0% | 89.2% | 89.3% | ||
7 | Hebei | 83.3% | 81.9% | 85.6% | 84.4% | 85.5% | 84.3% | ||
8 | Qinghai | 93.2% | 84.6% | 93.6% | 85.2% | 93.8% | 86.8% | ||
9 | Fujian | 82.2% | 84.9% | 85.0% | 86.3% | 86.4% | 87.1% | ||
10 | Shandong | 91.3% | 88.9% | 90.6% | 88.9% | 91.9% | 90.2% | ||
11 | Liaoning | 88.9% | 86.3% | 90.9% | 89.0% | 91.8% | 89.8% | ||
12 | Tibet | 82.5% | 66.1% | 83.5% | 67.1% | 84.3% | 68.7% | ||
13 | Inner Mongolia | 85.5% | 80.0% | 87.1% | 81.0% | 87.5% | 82.1% | ||
14 | Anhui | 89.3% | 87.0% | 89.8% | 87.1% | 90.5% | 88.0% | ||
15 | Guangxi | 73.3% | 68.7% | 74.9% | 70.1% | 75.4% | 71.0% | ||
16 | Chongqing | 86.2% | 72.9% | 86.6% | 72.9% | 86.1% | 74.8% | ||
17 | Shaanxi | 89.2% | 84.8% | 90.3% | 84.8% | 91.3% | 86.2% | ||
18 | Ningxia | 91.6% | 79.0% | 92.0% | 79.3% | 92.2% | 79.4% | ||
19 | Beijing | 78.5% | 79.7% | 82.4% | 82.7% | 82.1% | 82.9% | ||
20 | Guizhou | 76.6% | 72.2% | 77.3% | 72.6% | 77.2% | 73.1% | ||
21 | Jilin | 86.4% | 85.2% | 87.9% | 86.5% | 89.3% | 88.6% | ||
22 | Hubei | 87.1% | 75.8% | 88.2% | 74.7% | 88.4% | 74.8% | ||
23 | Jiangsu | 85.4% | 77.5% | 85.3% | 77.4% | 86.2% | 80.1% | ||
24 | Henan | 86.8% | 80.6% | 87.9% | 82.0% | 87.8% | 82.5% | ||
25 | Heilongjiang | 88.4% | 80.6% | 88.8% | 82.3% | 89.6% | 82.3% | ||
26 | Taiwan | 91.0% | 75.9% | 91.2% | 78.4% | 90.8% | 79.8% | ||
27 | Jiangxi | 77.0% | 70.1% | 79.4% | 72.8% | 81.1% | 72.7% | ||
28 | Zhejiang | 85.8% | 85.2% | 86.8% | 85.6% | 87.9% | 86.3% | ||
29 | Tianjin | 85.1% | 76.7% | 87.3% | 78.6% | 87.1% | 79.2% | ||
30 | Hunan | 80.4% | 74.2% | 82.0% | 74.9% | 81.1% | 72.9% | ||
31 | Hainan | 81.6% | 81.1% | 84.4% | 82.8% | 86.2% | 85.7% | ||
32 | Shanghai | 81.6% | 87.5% | 88.3% | 91.1% | 88.2% | 87.7% | ||
33 | Hong Kong | 70.6% | 23.5% | 75.0% | 40.0% | 73.7% | 40.0% | ||
Note: The number of earthquake events gradually decreases from Sichuan to Hong Kong. The block pickers for Yunnan, Hebei, Beijing, Hunan, Shanghai and Hong Kong are TP, NC, NC, SC, NC and SC, respectively. |