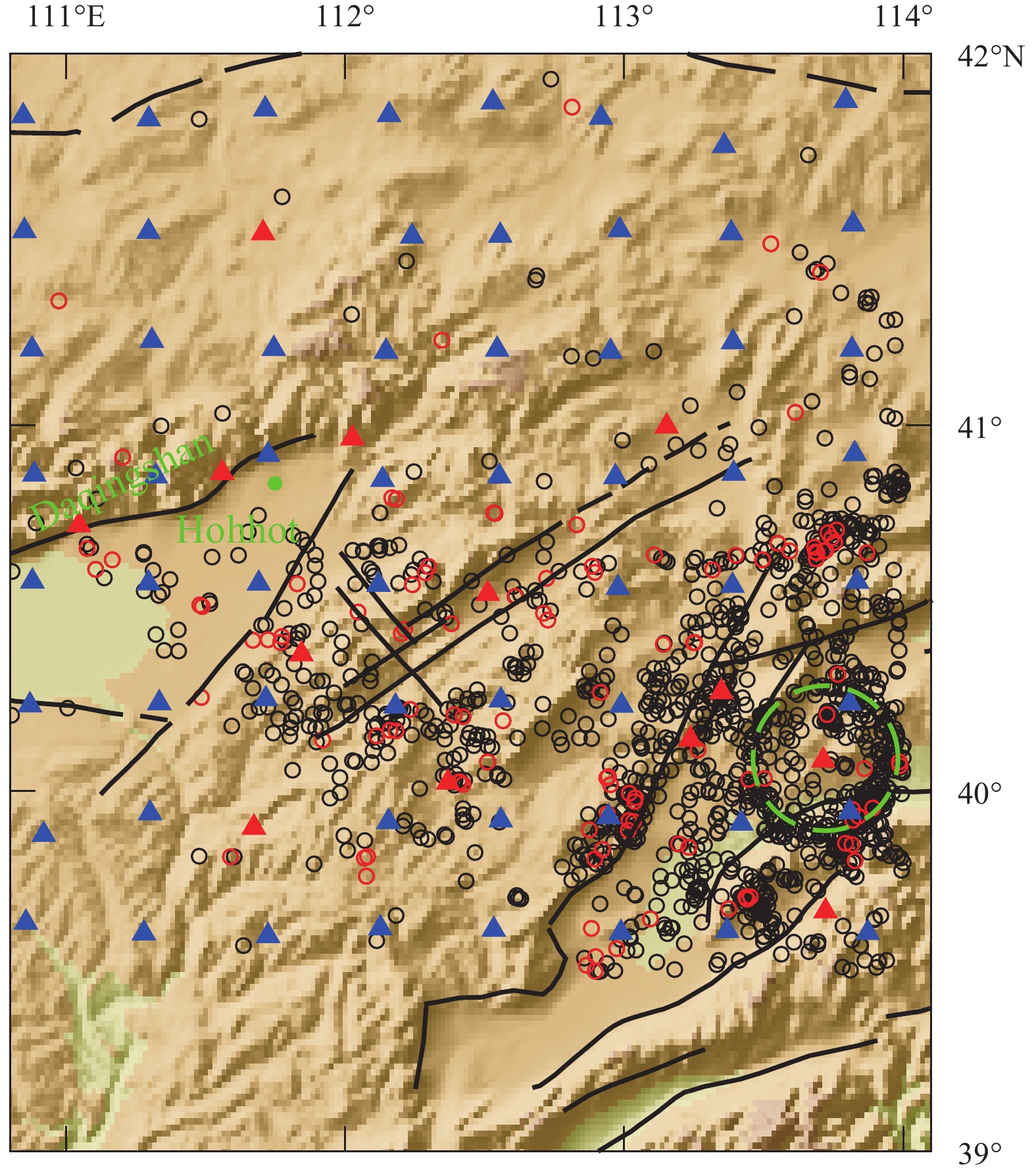
Citation: | Long Zhang, Lihua Fang, Weilai Wang, Zuoyong Lv (2020). Seismic phase picking in China Seismic Array using a deep convolutional neuron network. Earthq Sci 33(2): 72-81. DOI: 10.29382/eqs-2020-0072-03 |
Seismic phase picking plays an important role in earthquake location (Fang et al., 2015, Shelly, 2020) and illuminates the Earth’s interiors via body wave tomography (Xin et al., 2019, Share et al., 2019). In recent years, more and more large seismic arrays are deployed in China, United States and many other regions, it’s very difficult for human experts to pick all the phase data timely. Manual picking is commonly considered as the most accurate way over all existing methods but time consuming. Besides, their consistency depends on the experience and preference of human experts. Although some automatic picking algorithms has been developed, such as short-term average over long-term average (STA/LTA) algorithm (Allen, 1982) and Auto Regression- Akaike Information Criterion (AR-AIC) (Sleeman and Van Eck, 1999), their accuracy relies on the user’s experience and their efficiency is dependence of data length. These traditional picking methods have not been alternatives of human experts completely.
Rapidly developing machine learning technologies show significant advantages over traditional phase picking methods. The traditional methods just use a small part of manually preliminarily-defined features for arrival-time point, while machine learning technologies could extract more rich features automatically from a large labeled training dataset. The trained models especially generated from deep convolutional neuron networks (CNN) has been validated in some testing datasets which are from different regions and magnitude range (Ross et al., 2018, Zhao et al., 2019, Zhou et al., 2019, Zhu et al., 2019).
The northeastern margin of Ordos block is one of the high seismicity regions in China. The routine catalog of this region is produced from a sparse regional seismic network with an average station space of 100 km. Because of the sparse station distribution, some small earthquakes are missed or just recorded at one or two stations. It is hard to determine the precise location of these earthquakes only based on the regional network. To investigate the seismicity and velocity structures of this region, ChinArray-Phase III deployed 60 temporary broadband stations in this area from August 2016 to January 2019.
Previous studies with CNN focused on earthquake detection with permanent seismic stations. In this study, we attempt to investigate the validity of automatic phase picking method in a temporary seismic array with high background noise. Firstly, P and S wave arrival times are picked by PickNet, one representative CNN model. Then, one popular traditional picking method, AR pick, is also applied to the same testing dataset. Secondly, the picking errors of PickNet and AR pick are compared. Finally, earthquakes in the study area are relocated based on picks from PickNet.
In this study, the trained PickNet models are applied to pick arrival times of P and S phases. The PickNet network is developed to pick more phases for a dense array when the catalog is known (Wang et al., 2019). It can be considered as a combination of the classical CNN, a modified VGG-16 model (Simonyan and Zisserman, 2014), and a Rich Side‐output Residual Networks (Liu et al., 2017). ~460,000 first P and ~280,000 first S slices of High sensitivity Seismic Network (Hi-net) records are trained to obtain the optimal models for P and S arrival times picking, respectively (Wang et al., 2019). The trained PickNet models have an accuracy of 85.4% and 60.8% for P and S-wave error bin of 0.1 s in a testing dataset which consists of ~234,600 seismograms. Besides, the arrival times picked by PickNet is 7.1 and 10.7 times of manually picked P and S phases, respectively, which is a significant increase of reliable data for travel time tomography.
The study region is located in the range of 110.8°E–114°E, 39.5°N–42°N (Figure 1). A temporary array, a part of ChinArray-III (X3), is deployed in this region from August 2016 to January 2019. The earthquake catalog during this period determined by the regional seismic network is accessed (http://10.5.160.18/console/exit.action). In total, there are 1,520 earthquakes recorded by regional seismic network. Among them, P and S arrival times of the events occurring from January 2017 to August 2017 with local magnitude (ML) larger than 1 are manually picked (Liu et al., 2020).
Preprocessing is required before picking arrival time. 210-s-long event waveforms are accessed based on earthquake origin time (–30–180 s). As recommended by Wang et al. (2019), these event waveforms are then cut to 12-s-long and 16-s-long slices and centered at their theoretical arrival times for picking the first P and S-phase arrival times, respectively. Theoretical arrival times are calculated by TauP (Crotwell et al., 1999) and AK135 velocity model (Kennett et al., 1995). Note that the crustal depth is adjusted to 45 km according to Wang et al. (2017)’s inversion result based on the receiver functions. Same as Wang et al. (2019)’s preprocessing, the P-wave slices are from Z component while the S-wave slices are from R and T components, because the models for P and S picking are trained separately. Finally, we remove linear trend and normalize all the slices before picking.
To validate the accuracy of PickNet, P and S-wave arrival times picked by PickNet and human experts are compared. Note that only the arrival times at stations with the epicentral distance smaller than 200 km are compared. Since a relatively high signal-to-noise ratio (SNR) around the picks in this distance range, arrival times are usually easy to pick manually. 44% first P and 47% S-phase arrival times are missed (Figure 2). If this part of arrival times picked by the PickNet models are accurate, it will extremely enrich the dataset for earthquake relocation and travel-time tomography. There are only 1.5% P and 1.9% S arrival times are missed by PickNet.
The arrival times picked by both PickNet and human experts (dark blue part in Figure 2) are carefully compared. Picking error, which is defined as the arrival time manually picked minus those picked by automatic methods, is calculated. Figure 3a and 3c are the picking error histograms of P and S wave using PickNet, respectively. To have a better evaluation of picking accuracy, one traditional automatic arrival-time picking method is also applied to these event waveforms. The combination of AR-AIC and STA/LTA algorithm is developed as “AR pick” (Akazawa, 2004) and implemented in ObsPy (Beyreuther et al., 2010), which has been widely used in arrival-time pickings (Akram and Eaton, 2016; Zhu and Beroza, 2019). The parameters of AR pick are set as follows. The frequency range of a band-pass filter is 1–20 Hz. The lengths of LTA and STA for the P arrival are 1 s and 0.1 s, while for the S arrival are 2 s and 0.2 s, respectively. Figure 3b and 3d are the picking error histograms of P and S wave using AR pick, respectively. The mean value and standard deviation of the picking error are also shown in each subplot. It is well known that the mean value shows the systematical deviation of one picking criterion while the standard deviation represents the picking precision. Compare to the AR pick, PickNet has a better picking precision due to the smaller standard deviation.
The picking errors of PickNet models and AR pick are compared quantitatively in five main error bins. Table 1 shows the number and percentage of picking errors. Compared with the traditional automatic picking algorithm, significantly improvement is obtained by PickNet in each error bin, especially in the 0.1 s and 0.5 s error bins for P and S wave, respectively, which are concerned by the researchers who investigate crustal velocity structures using the body wave travel time tomography method and by the geologists who explore the shallow fault structure using earthquake relocation technologies.
Error | ±0.1 s | ±0.2 s | ±0.5 s | ±1 s | ±2 s | ||
P wave (1226) | PickNet | Number | 1023 | 1113 | 1193 | 1216 | 1223 |
Percentage | 83.44% | 90.78% | 97.31% | 99.18% | 99.76% | ||
AR pick | Number | 755 | 869 | 963 | 986 | 1013 | |
Percentage | 61.58% | 70.88% | 78.55% | 80.42% | 82.63% | ||
S wave (1181) | PickNet | Number | 719 | 887 | 1051 | 1101 | 1127 |
Percentage | 60.88% | 75.11% | 88.99% | 93.23% | 95.43% | ||
AR pick | Number | 162 | 249 | 407 | 567 | 787 | |
Percentage | 13.72% | 21.08% | 34.46% | 48.01% | 66.64% | ||
Note: The number of arrivals picked by both PickNet and human experts are shown in the 1st column |
Seismograms of each earthquake and picked arrival times for P and S wave are plotted. Figure 4 shows an example of the comparison of picked arrival times of a ML 1.2 earthquake. Almost all the arrival times picked by PickNet have a similar result to human experts. Figure 5 shows several seismograms that the arrival times were missed by PickNet. It is reported that SNR has an influence on picking of PickNet (Wang et al., 2019). The possible reason of seismic phase missing in Figure 5 is the poor data quality.
The picking efficiency has been significantly improved. For one experienced human expert, picking 20 earthquakes where each event is recorded in an average of 20 stations reaches to his upper limit per day. If the signal windows are prepared well, picking 1,520 earthquakes will just cost ~30 s by PickNet, which benefits from one Tesla V100 graphic processing units (GPU), while will cost ~3 minutes by AR pick. It suggests that the picking efficiency of PickNet is improved.
Earthquake relocation is performed to investigate the picking accuracy and precision of PickNet. To produce high-precision hypocenter locations, we first applied HypoSAT (Schweitzer, 2001) to obtain the absolute location of the 1,252 earthquakes, each of which has six phases at least. Second, the picks with the location residual smaller than 0.6 s and phase number greater than 4 are selected. 847 earthquakes meet this criterion and then are considered as the input for double-difference location. Then we perform HypoDD (Waldhauser and Ellsworth, 2000), one widely used relative location method, to get more precise locations. Because of a large study area and a sparse earthquake distribution, there are 522 weakly linked earthquakes identified by HypoDD. Finally, the precision location of 573 earthquakes are obtained after relocation. The average relocation errors in NS, EW and the depth are 0.38 km, 0.36 km and 0.51 km, respectively. The average travel time residual is 0.15 s. They are of the same order of magnitude in comparison with other researches on aftershock sequence relocation (Fang et al., 2014, Wang et al., 2014). It suggests that the high-precision arrival times picked by PickNet can be used for earthquake relocation.
Compare with the original locations, the relocated earthquakes concentrate on fault traces in surface and depth. The earthquake distribution before and after relocation is shown in Figure 6. The relocated earthquakes concentrate on fault traces, especially on Kouquan fault (F4 in Figure 6). Besides, the fake circular earthquake distribution in Figure 1 is disappeared. One profile A-A’ is designed to investigate the earthquake distribution in depth. Earthquakes in 10 km across the profile A-A’ are projected. Figure 7 shows the cross section of profile A-A’ before and after relocation. Benefiting from the high-quality travel time dataset from PickNet, a high-precision earthquake relocation is obtained. Compared with the human processing routine catalog, the number of earthquakes in profile A-A’ increases from 139 to 152 after relocation. It indicates relocated earthquakes are more concentrated. In Figure 7a, the Datong basin can not be constrained well by the routine catalog. After relocation, earthquakes concentrate on the bound faults, such as Kouquan fault (F4), which is the western boundary of Datong basin. Relocation indicates Kouquan fault is a high dip-angle fault. It is in good agreement with the conclusion that Kouquan fault is a right-lateral strike-slip fault with the dip angle of 60°–80°, which is drawn by using geological techniques (Deng and Xu, 1995, Xu et al., 2011). Relocation also shows the dip-angle changes in different section of Kouquan fault. In Figure 6, the earthquake projection of northern section of Kouquan fault on the surface is shown in a green dashed line. The gap between the projection and surface trace indicates the dip-angle switches from ~90° at central section to ~70° at northern section. The earthquakes within basins in shallow depth is rare. It may correspond to a weakening stiffness for the shallow crust and thus difficult to accumulate stress. The relocation also shows that the focal depth is as deep as 12 km in Huhe depression, which is shallower than that of Datong basin (~16 km). It is in good agreement with Cai et al. (2014)’s result, who use the catalog from 2008 to 2012.
In this study, the validity of automatic phase picking method in a dense seismic array is investigated. Almost all the phases in the bulletin are picked with high precision. Their picking error in 0.1 s bin for P and 0.5 s bin for S are acceptable. However, some examples with large error need to analyze further (Figure 8). One reason of large picking error may be due to low SNR in a long distance (e.g. P wave in station X3.14801). Another reason is the complexity of the seismogram (e.g. S wave in station X3.14802). A rich training dataset could improve the performance of the CNN, because much complicated feathers of seismograms have been learned while training. Two possible ways are capable of expanding the training dataset. One is to collect large amount of arrival times from different regions with wide magnitude and epicentral distance ranges. The other is to apply the data augmentation technology (e.g. to add some noises to the training samples).
The location residual can be considered as one powerful tool for the selection of arrival time from automatic picking algorithms. In the most researches on phase picking from the automatic algorithms, especially for the popular machine learning methods, there is no an appropriate way to evaluate the more picked arrival times. In this study, we suggest to use the location residual to evaluate them. Figure 9a shows raw travel time curves from PickNet. Different from the concentrated manually picked travel time distribution, that from the PickNet is much wider, which shows a large variation. It is possible that part of arrival times from PickNet are not too much accurate. A selection should be done to have a strict limit before using these arrival times. The travel time with location residual of HypoSAT smaller than 0.6 s is selected (Figure 9b), which is more concentrated. Then, these selected travel times are input to HypoDD to determine the relative location of earthquakes. The relocation error in three components (N, E, Z) and travel time residual decrease from 0.63 km, 0.60 km, 0.79 km and 0.21 s to 0.38 km, 0.36 km, 0.51 km and 0.15 s, respectively. Besides, the signal-to-noise ratio (SNR) is also calculated for the selected and dropped phases. Here, SNR is defined as the ratio of the root of sum of squared amplitudes in the range of 2 s after to 2 s before the target pick. The SNR for P-wave is determined from Z component of the sliced window, while the SNR for S is the average of that from R and T component of the sliced windows. The percentage of SNR larger than 2 for the selected phases (29.8%) is nearly twice of that for the dropped phases (15%). It suggests that a higher precision location result is obtained when using the selected travel-time data. It also indicates some large-error arrival times are excluded and the quality of travel-time dataset is improved after the selection.
The SNR and epicentral distance for manually missed picks are calculated. Figure 10 shows that for most of the manually missed picks, SNR is smaller than 2 or distance is larger than 100 km. It suggests that human experts can not identify the picks for low-quality waveforms while PickNet can do it. 862 manually missed picks are left after phase selection and then used by HypoDD, which account for 41.5% of the manually missed picks (981 P and 1095 S in Figure 2). To achieve a reliable location, a sufficient number of high-precision picks and a good station distribution are necessary. In this study, high-quality manually missed picks contributes to expanding the travel-time dataset for earthquake relocation.
To demonstrate the power of PickNet, the picks from AR pick are also used to locate the same earthquakes. Before the relative location, phase selection is also performed. There are 722 earthquakes and 8,900 picks after the selection for AR pick, which is smaller than 847 earthquakes and 15,722 picks for PickNet. It indicates the PickNet could provide reliable picks for earthquake location.
The earthquakes recorded by a single station is usually hard to be precisely located due to sparse regional seismic network. The small earthquake recorded at single station of a sparse regional seismic network can be called as single-station earthquake. In the catalog of this study, single-station earthquakes account for about one quarter. It is usually hard to locate the single-station earthquakes precisely. However, this kind of earthquake usually indicates a tiny stress-release process and helps refine the knowledge of fault structures. Therefore, a relocation should be performed for them. Benefiting from the implementation of dense arrays, the single-station earthquakes are also recorded at several stations. The arrival times of seismic phases in these stations play an important role in relocation. We proposed a flowchart to determine the accurate location of the single-station earthquake via dense portable seismic array and phase arrival picked by PickNet. There are 349 single-station earthquakes with 4,894 P and S arrivals among the selected 1,252 earthquakes. Our method expands the arrival-time dataset and improve the location accuracy of single-station earthquake.
Some conclusions can be drawn based on the above disscussion:
1) Compare with the traditional automatic methods, picking accuracy of CNN is significantly improved and acceptable. More importantly, manually missed arrival times can be picked. The picking efficiency is also greatly raised in comparison with human experts. It suggests that automatic methods, especially the CNN method, can be applied to pick seismic phases for a large dense array.
2) The current PickNet algorithm has some limitations. For example, it only can be used when the catalog is provided. The development should be focused on improving the picking accuracy and processing continuous waveforms in the future.
3) The automatically picked arrival times should be selected before performing earthquake relocation or tomography inversion. The absolute location residual is suggested to be a useful indicator for travel time selection. In our case, the relocation error in three components and travel-time residual decrease a lot when using the selected travel-time data.
4) A flowchart of precisely locating the single-station earthquakes is proposed. For a given single-station catalog produced from a sparse regional network, the arrival times of seismic phases in the records of a dense array in the same region could be picked by the machine learning methods. Then the widely used earthquake-location methods are performed to relocate them precisely with the picked arrival times. Benefiting from these precise picks, the original arrival-time dataset is enriched and the catalog precision is improved.
This study was financially supported by National Key R&D Program of China (No.2018YFC1504103), the National Natural Science Foundation of China (No. 41774067) and the Special Fund of the Institute of Geophysics, China Earthquake Administration (Nos. DQJB19B05 and DQJB20X07). The authors would like to thank Dr. Jian Wang for sharing their trained PickNet models. Two anonymous reviewers are acknowledged for their constructive comments, which helped improve this article. Waveform data for this study are provided by Data Management Center of China Seismic Array (http://www.chinarraydmc.cn). The arrival-time dataset is provided by Dr. Yaning Liu.
Akazawa T (2004). A technique for automatic detection of onset time of P-and S-phases in strong motion records. Proceedings of the 13th World Conference on Earthquake Engineering. Vancouver B C, Canada, p786
|
Akram J and Eaton DW (2016) A review and appraisal of arrival-time picking methods for downhole microseismic data. Geophysics 81(2): KS71–KS91 doi: 10.1190/geo2014-0500.1
|
Allen R (1982) Automatic phase pickers: Their present use and future prospects. Bull Seismol Soc Amer 72(6B): S225–S242
|
Beyreuther M, Barsch R, Krischer L, Megies T, Behr Y and Wassermann J (2010) ObsPy: A Python toolbox for seismology. Seismol Res Lett 81(3): 530–533 doi: 10.1785/gssrl.81.3.530
|
Cai Y, Wu JP, Fang LH, Wang WL and Huang J (2014) Relocation of the earthquakes in the eastern margin of Ordos block and their techtonic implication in the transition zones of extensional basin. Chin J Geophys 57(4): 1079–1090 (in Chinese with English abstract)
|
Crotwell HP, Owens TJ and Ritsema J (1999) The TauP Toolkit: Flexible seismic travel-time and ray-path utilities. Seismol Res Lett 70(2): 154–160 doi: 10.1785/gssrl.70.2.154
|
Deng QD and Xu XW (1995) Segmentation Study of Active Faults in the Shanxi Fault-Depression Basin Belt. In: Institute of Geology, State Seismology Bureaueded. Recent Crustal Movement (6). Beijing, Seismological Press, pp.225–242 (in Chinese)
|
Fang L, Wu J, Wang W, Du W, Su J, Wang C, Yang T and Cai Y (2015) Aftershock observation and analysis of the 2013 MS 7.0 Lushan earthquake. Seismol Res Lett 86(4): 1135–1142 doi: 10.1785/0220140186
|
Fang LH, Wu JP, Wang WL, Lv ZY, Wang C, Yang T and Zhong SJ (2014) Relocation of the aftershock sequence of the MS6.5 Ludian earthquake and its seismogenic structure. Seismol Geol 36(4): 1173–1185 (in Chinese with English abstract)
|
Kennett BL, Engdahl E and Buland R (1995) Constraints on seismic velocities in the Earth from traveltimes. Geophys J Int 122(1): 108–124 doi: 10.1111/j.1365-246X.1995.tb03540.x
|
Liu C, Ke W, Jiao J and Ye Q (2017). Rsrn: Rich side-output residual network for medial axis detection. Proceedings of the IEEE International Conference on Computer Vision, Venice, Italy, pp.1739–1743
|
Liu YN, Wu JP, Wang WL and Yang T (2020) Travel time tomography for 3D crustal P-wave velocity structure in Ordos and its adjacent area. in preparation
|
Ross ZE, Meier MA, Hauksson E and Heaton TH (2018) Generalized seismic phase detection with deep learning. Bull Seismol Soc Amer 108(5A): 2894–2901 doi: 10.1785/0120180080
|
Schweitzer J (2001) HYPOSAT–An enhanced routine to locate seismic events. Pure Appl Geophys 158(1-2): 277–289
|
Share P-E, Guo H, Thurber CH, Zhang H and Ben-Zion Y (2019) Seismic imaging of the southern California Plate boundary around the South-Central Transverse Ranges using double-difference tomography. Pure Appl Geophys 176(3): 1117–1143 doi: 10.1007/s00024-018-2042-3
|
Shelly DR (2020) A high-resolution seismic catalog for the initial 2019 Ridgecrest earthquake sequence: Foreshocks, aftershocks, and faulting complexity. Seismol Res Lett 91(4): 1971–1978, doi: https://doi.org/10.1785/0220190309
|
Simonyan K and Zisserman A (2014) Very deep convolutional networks for large-scale image recognition. arXiv preprint arXiv: 1409.1556
|
Sleeman R and Van Eck T (1999) Robust automatic P-phase picking: An on-line implementation in the analysis of broadband seismogram recordings. Phys Earth Planet Interi 113(1-4): 265–275 doi: 10.1016/S0031-9201(99)00007-2
|
Waldhauser F and Ellsworth WL (2000) A double-difference earthquake location algorithm: Method and application to the northern Hayward fault, California. Bull Seismol Soc Amer 90(6): 1353–1368 doi: 10.1785/0120000006
|
Wang J, Xiao ZW, Liu C, Zhao DP and Yao ZX (2019) Deep learning for picking seismic arrival times. J Geophys Res 124: 6612–6624 doi: 10.1029/2019JB017536
|
Wang W, Wu J, Fang L, Lai G and Cai Y (2017) Sedimentary and crustal thicknesses and Poisson's ratios for the NE Tibetan Plateau and its adjacent regions based on dense seismic arrays. Earth Planet Sci Lett 462: 76–85 doi: 10.1016/j.jpgl.2016.12.040
|
Wang W, Wu JP, Fang LH and Lai GJ (2014) Double difference location of the Ludian MS6.5 earthquake sequences in Yunnan Province in 2014. Chin J Geophys 57(9): 3042–3051 (in Chinese with English abstract)
|
Xin HL, Zhang HJ, Kang M, He RZ, Gao L and Gao J (2019) High-resolution lithospheric velocity structure of continental China by double-difference seismic travel-time tomography. Seismol Res Lett 90(1): 229–241 doi: 10.1785/0220180209
|
Xu W, Liu XD and Zhang SM (2011) Late Quaternary faulted landforms and determination of slip rates of the middle part of Kouquan fault. Seismol Geol 33(2): 336–346
|
Zhao M, Chen S, Fang LH and David AY (2019) Earthquake phase arrival auto-picking based on U-shaped convolutional neural network. Chin J Geophys 62(8): 3034–3042 (in Chinese with English abstract)
|
Zhou Y, Yue H, Kong Q and Zhou S (2019) Hybrid event detection and phase-picking algorithm using convolutional and recurrent neural networks. Seismol Res Lett 90(3): 1079–1087 doi: 10.1785/0220180319
|
Zhu L, Peng Z, McClellan J, Li C, Yao D, Li Z and Fang L (2019) Deep learning for seismic phase detection and picking in the aftershock zone of 2008 MW7.9 Wenchuan Earthquake. Phys Earth Planet Interi 293: 106261 doi: 10.1016/j.pepi.2019.05.004
|
Zhu W and Beroza GC (2019) PhaseNet: A deep-neural-network-based seismic arrival-time picking method. Geophys J Int 216(1): 261–273
|
Error | ±0.1 s | ±0.2 s | ±0.5 s | ±1 s | ±2 s | ||
P wave (1226) | PickNet | Number | 1023 | 1113 | 1193 | 1216 | 1223 |
Percentage | 83.44% | 90.78% | 97.31% | 99.18% | 99.76% | ||
AR pick | Number | 755 | 869 | 963 | 986 | 1013 | |
Percentage | 61.58% | 70.88% | 78.55% | 80.42% | 82.63% | ||
S wave (1181) | PickNet | Number | 719 | 887 | 1051 | 1101 | 1127 |
Percentage | 60.88% | 75.11% | 88.99% | 93.23% | 95.43% | ||
AR pick | Number | 162 | 249 | 407 | 567 | 787 | |
Percentage | 13.72% | 21.08% | 34.46% | 48.01% | 66.64% | ||
Note: The number of arrivals picked by both PickNet and human experts are shown in the 1st column |